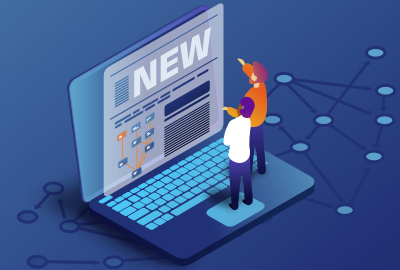
New BayesiaLab 11.4
Learn about the new features in Hellixia, BayesiaLab's native GeniAI integration.
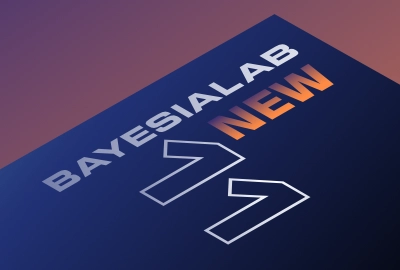
BayesiaLab
The Leading Desktop Software for Research, Analytics, & Reasoning with Bayesian Networks
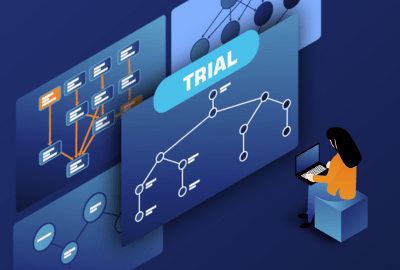
BayesiaLab Trial
Explore all of BayesiaLab's features in a free 30-day trial.
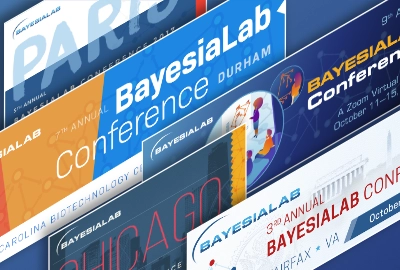
2025 BayesiaLab Conference
Join the BayesiaLab Conference on March 6–7 at Florida Atlantic University in Boca Raton, Florida, and Learn from Leading Experts.
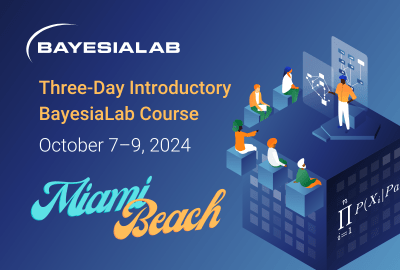
New BayesiaLab Courses
Learn all about Bayesian networks in the highly-acclaimed BayesiaLab Courses coming up in Miami Beach in October.
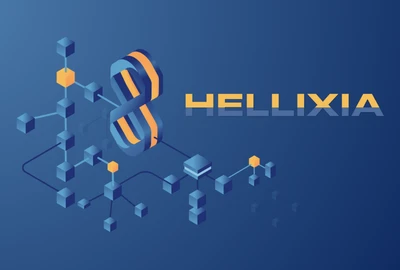
Hellixia
BayesiaLab's new subject matter assistant powered by GenAI.
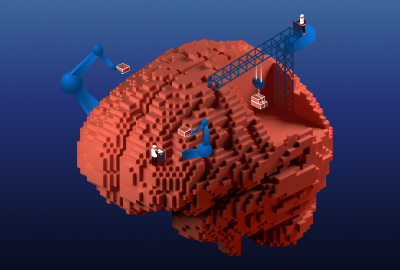
BRICKS
A probabilistic relational modeling framework.
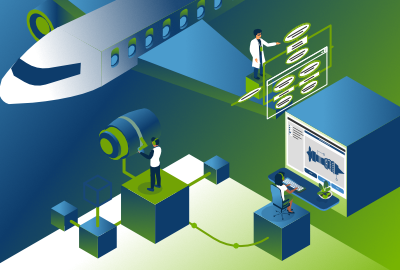
BEST
A Bayesian expert system for troubleshooting.
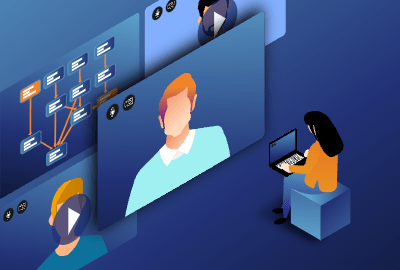
Upcoming BayesiaLab Events
Courses, seminars, & events around the world.
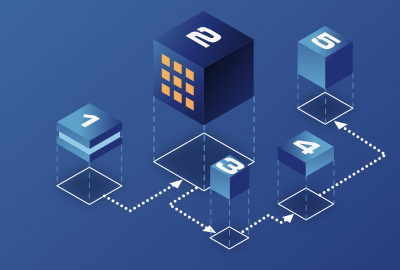
BayesiaLab User Guide
1,500+ Pages of documentation.
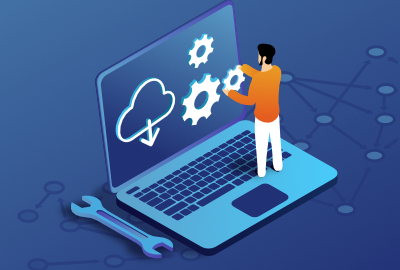
BayesiaLab Installation Guide
Set up BayesiaLab on your Windows, Mac, or Linux/Unix computer.
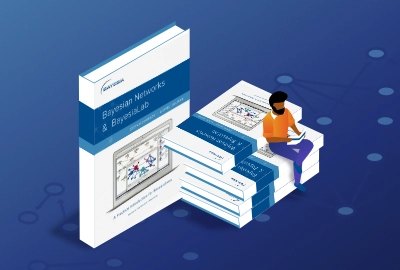
The BayesiaLab Book
E-Book: Bayesian Networks & BayesiaLab — A Practical Introduction for Researchers.
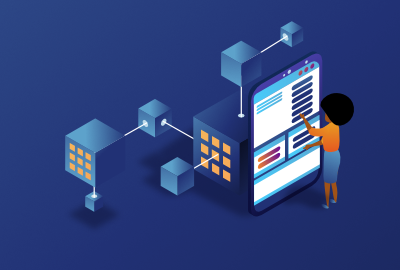
BayesiaLab WebSimulator
Publish your BayesiaLab models as a WebSimulator.
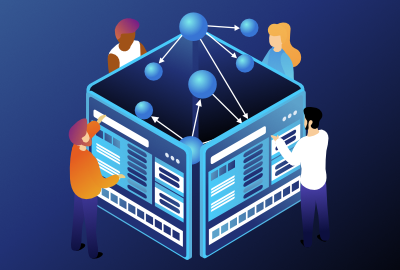
Bayesia Expert Knowledge Elicitation Environment (BEKEE)
Construct Bayesian networks with expert knowledge.
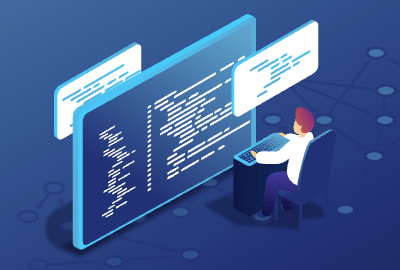
Bayesia Engine API
Learn and deploy Bayesian networks programatically.
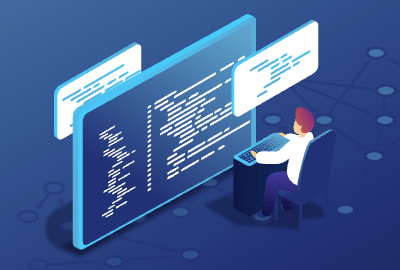
Get Software link
Regester your license and get the software.