Most Relevant Explanations
Context & Definition
The Most Relevant Explanations (MRE) feature uses a genetic algorithm for searching partial instantiations of sets of variables that maximize the Generalized Bayes Factor (GBF) as the best explanations for a given set of evidence.
This approach is primarily based on an article written by Yuan, C. et al. (2011), "Most Relevant Explanations in Bayesian Networks (opens in a new tab)", Journal of Artificial Intelligence Research, pp. 309-352.
The Weight of Evidence (WoE), which is the logarithm of the Generalized Bayes Factor (GBF), dates back to work by Alan Turing in World War II (see Good, I. J. (1985), "Weight of Evidence: A Brief Survey (opens in a new tab)", Bayesian Statistics 2, pp. 249-270, for more general discussion of history and concepts):
where
- E represents the current set of evidence (any type of evidence on any number of variables) for which we are seeking concise and precise explanations, and
- H is the hypothesis consisting of hard evidence on a subset of variables
where is the negation of , and denotes the odds:
As we can see, the GBF is expressed with two equivalent terms:
a likelihood ratio. an odds ratio.
- Even though they are strictly equivalent, the Likelihood Ratio is a natural interpretation of the GBF if the explanation H you are looking for belongs to the Ancestors of E in a causal Bayesian network.
- Note that a Bayesian network is causal if you can assert that all arc directions are oriented from cause to effect.
- If your Bayesian network is not causal, you can interpret the GBF as an Odds Ratio.
- You can also consider the GBF an Odds Ratio if the explanation H you are searching for does not belong exclusively to the Ancestors of E.
Usage
- To start the Most Relevant Explanations function, select Main Menu > Report > Evidence > Most Relevant Explanations.
- This function requires at least one observed node, i.e., at least one piece of evidence must be set.
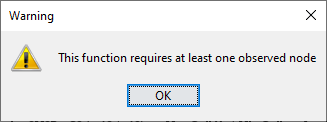
- In a new window, you can now define the options for searching for the Most Relevant Explanations of your current set of evidence E.
- This window features five main sections, which we discuss in detail below.
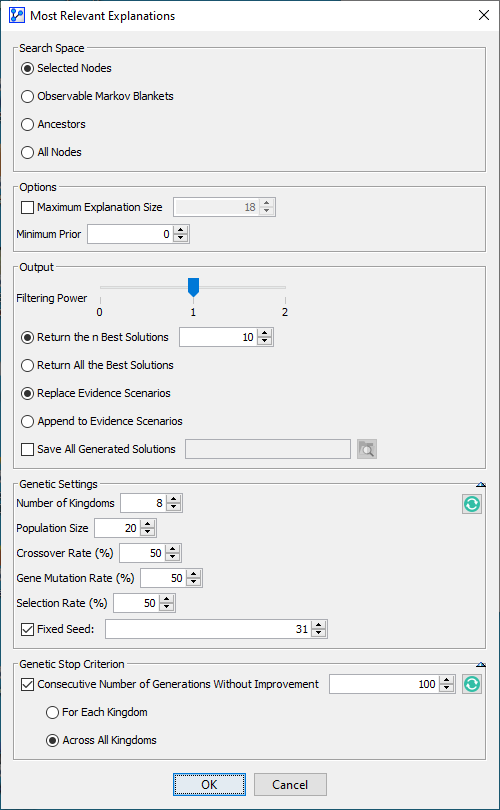
Search Space
- In the Search Space section, you can define the nodes to be included in the search. You have 4 options:
- The Selected Nodes.
- The node belonging to the Observable Markov Blanket(s) of evidence E.
- The Ancestors of E. The Ancestor option is only relevant and useful if your network is causal.
- All Nodes.
- The nodes that belong to the currently set evidence E and those that are Not Observable are automatically excluded from the search.
Output
There are different options available for the output, i.e., for the Report and the Evidence Scenario File:
- Filtering Power:
- 0: All solutions are reported, i.e., none are removed by filtering.
- 1: All Strongly Dominated solutions are excluded from the report. An explanation is strongly dominated by the dominant explanation , if .
- 2: The **Strongly Dominated **solutions and Weakly Dominated solutions are excluded from the report. An explanation is weakly dominated by the dominant explanation .
- Return the n Best Solutions: returns only a subset of filtered solutions, i.e., only the n best explanations.
- Return All the Best Solutions: returns all filtered solutions.
- Replace Evidence Scenarios: replaces your current Evidence Scenarios with the returned explanations.
- Append to Evidence Scenarios: adds the returned explanations to your Evidence Scenario File.
- Save All Generated Solutions: saves all unfiltered explanations to a text file you specify.
Genetic Settings
The BayesiaLab's Most Relevant Explanations (MRE) feature is using a genetic algorithm to search for the best explanations. This genetic algorithm can be customized:
- Number of Kingdoms: number of populations that evolve independently.
- Population Size: number of individuals/solutions per population.
- Crossover Rate: the probability for the individuals of the worst kingdom to be generated by crossing the genes of the best individuals of two randomly selected kingdoms.
- Gene Mutation Rate: the probability to mute a gene of an individual.
- Selection Rate: the probability for an individual to be selected to breed the new generation.
- Fixed Seed: as the genetic algorithm is stochastic, using a fixed seed allows you to reproduce your results when running multiple iterations.
- Clicking reverts all settings to the default values.
- The icons in the upper right corner allows you to show and hide the options panel respectively.
Genetic Stop Criterion
- The genetic algorithm employed here is a so-called anytime algorithm, which will keep running unless it is stopped.
- You can stop the algorithm manually by clicking theicon in the lower-left corner of the Graph Window.
- Alternatively, you can define a stop criterion based on the consecutive number of generations without any improvement. The number of generations without improvement can be calculated at the kingdom level or across all kingdoms.
- Clicking reverts all settings to the default values.
- The icons in the upper right corner allows you to show and hide the options panel respectively.
Report Window
- Upon click OK at the bottom of the Settings Window, a new report window opens up that features the Most Relevant Solutions.
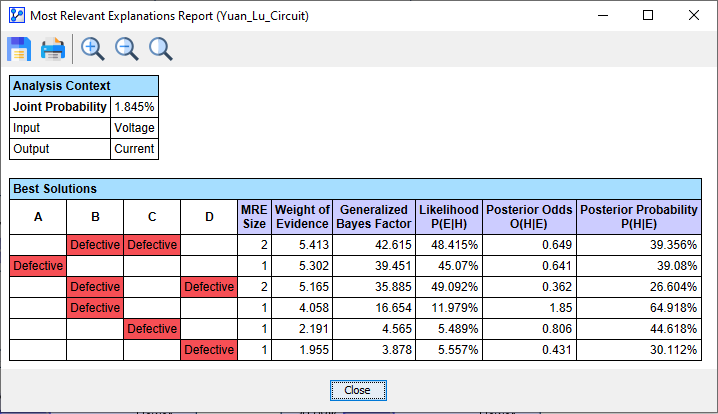
- We defer a detailed discussion of the report to examples, which feature a complete workflow. See the links to the examples below.