Dynamic Bayesian Networks
Graphical Representation
You can use a temporal dimension in the context of a static Bayesian network i.e. by "unrolling" the network to the desired number of time steps (i.e. by duplicating the network for each time step, as illustrated in the screenshot below for four time steps). However, this solution is only feasible for a limited number of time steps.
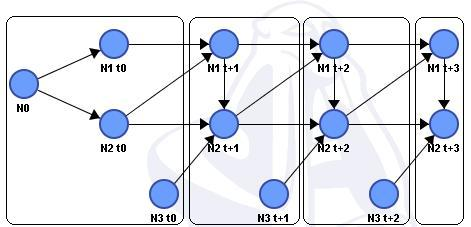
A Dynamic Bayesian Network provides a much more compact representation for such stochastic dynamic systems. The compactness is based on the following assumptions:
- The process is Markovian, i.e. the variables of time step t depend on a set of variables that belong to a limited set of former time steps (typically only on the previous time step t-1, i.e. a first-order Markovian assumption).
- The system is time invariant, i.e. the probability tables do not evolve as a function of time. This last assumption is partially relaxed in BayesiaLab as the Time Variable makes it possible to modify the probability distributions based on the value of the current time step with user-defined equations.
Given a first-order Markovian assumption, it is possible to represent such a system with only two time slices. The screenshot below shows the same network as the unrolled network presented above, however, without any limitation with regard to the number of time steps. The first slice describes the initial network at time step t0, and the second one describes the temporal transitions t+1.
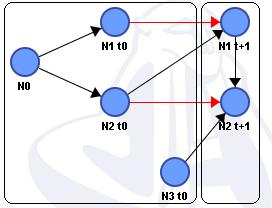
The specification of the t+1 slice is defined by means of Temporal Arcs (shown in red). A Temporal Arc indicates that the two connected nodes represent the same node at two consecutive time steps. As a result, the nodes belonging to the t+1 slice are the destination nodes of the Temporal Arcs.
For a Markovian assumptions of a higher order than one, the nodes of time slice t+1 are also linked to nodes belonging to time slices prior to time slice t0. However, Temporal Arcs can only be used to link nodes between time slices t0 and t+1.
The initial slice is particularly important because its synchronous arcs (connecting the nodes within the t0 slice, such as N0 -> N1 t0 and N0 -> N2 t0 in the example above) are removed after the first temporal inference. Then we have the common representation of a Dynamic Bayesian Network in which nodes of the t slice have no parents.
Inference
Inference in a Dynamic Bayesian Network is not as simple as with a static Bayesian network. BayesiaLab proposes two kinds of inference:
- Inference based on a Junction Tree, which yields exact inference for static networks, but returns approximate results for dynamic networks. In special cases, e.g. for the valve system shown below, the inference is exact because the valves are independent from each other. Similar to Bayesian Updating, obtaining exact results with dependent temporal nodes, it is necessary to qualitatively indicate the dependencies (without modifying the original conditional probability table to keep the possible marginal independence). Simply add arcs between these dependent nodes, as illustrated below.
- Inference based on Monte Carlo simulation (particle filtering), which yields approximate inference in the static and dynamic case. In both cases, the approximation is of the same order, i.e. the approximation is not related to the dependence of the nodes; rather, it is only due the randomness of the simulation.
Temporal Simulation
In Validation Mode, the toolbar has five new tools for temporal simulation:

- resets the network, i.e. the synchronous arcs and the probability tables of the t0 slice are reset. The network also resets after entering new probability distributions for the temporal parent nodes and when switching to Modeling Mode.
- time meter indicates the current value
of the time step. This meter can also be used to enter the time steps to be reached,
i.e. the length of the simulation. If this number is lower than the current value
of the time step value, the network will be reset. Otherwise, the simulation is
carried out from the current time step to the set number. The simulation can be
stopped by clicking on the red light in the status bar. If a Temporal Evidence
Scenario File is associated with the network, right-clicking on the index field
displays a list of the evidence sets. A click on any line in the list performs
the temporal simulation from the current index to the specified index, taking into
account the corresponding sets of evidence:
- simulate temporal step by temporal step.
- graphical view of the probability evolution of the Temporally-Spied Nodes, as shown below.
If a Temporal Evidence Scenario File is associated with the network, the corresponding evidence for each time step will be taken into account. By pressing the button located in the Monitor's toolbar, it is also possible to add a set of evidence for the current time step. Please note that setting the probability distribution of a node will change according to the chosen inference: fixed distribution with exact inference or computation of the corresponding likelihoods with approximate inference.
Sometimes a fixed probability distribution cannot be exactly achieved because the algorithm for computing the target distribution fails to converge. In this case, a warning is displayed, and a message is posted to the Console.
The Temporal Chart button displays the following window:
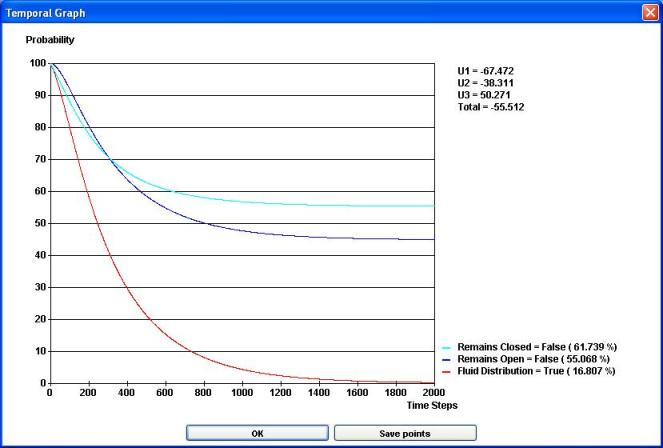
For each Spied State, the mean of the probability over the simulated period appears in the legend. If the network has utility nodes, the mean of the expected value of each utility node appears in the upper right corner, as well as the sum of these expected values.
A right-click on the graph brings up the Contextual Menu, which allows you to select the following:
- Show a relative view of the graph. The Y-axis shows the minimal and maximal values rather than the 0-to-100 scale, which is the default view.
- Print the graph.
- Copy the graph, which can then be pasted as an image or as a data array into external applications. Points can also be saved directly into a text file.