Herman Melville: Moby Dick (1851)
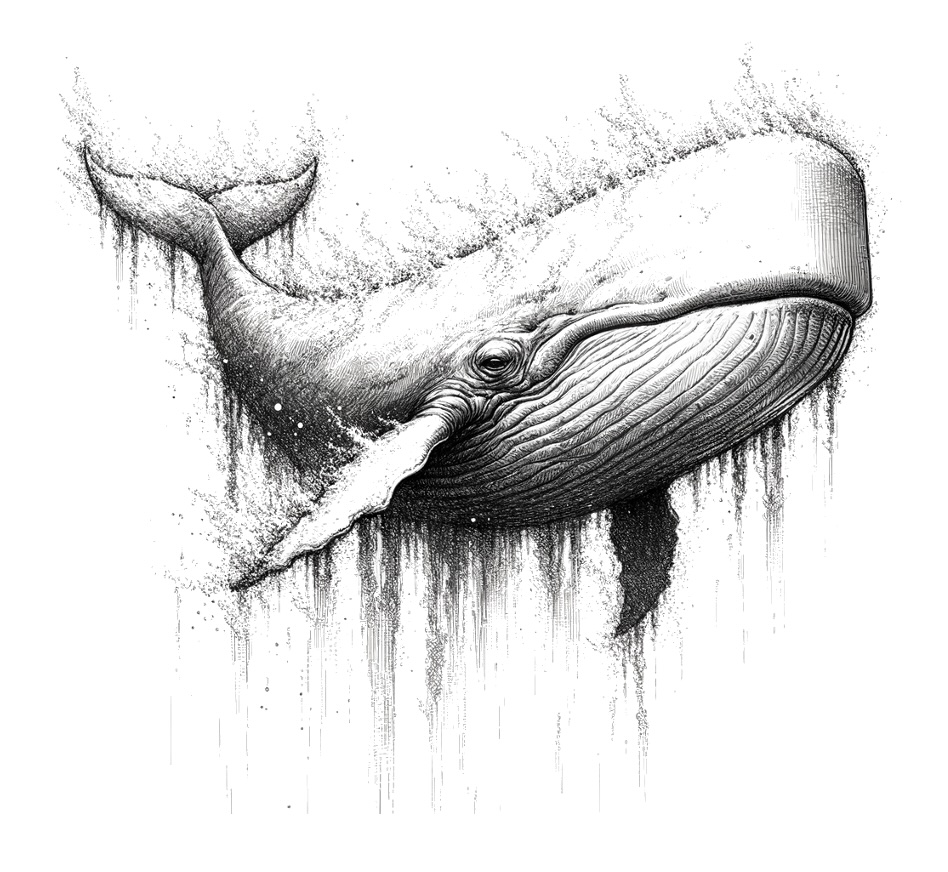
Navigating the Depths: A Multi-Faceted Analysis of "Moby Dick" Using Hellixia's Advanced Features
Herman Melville's Moby Dick, published in 1851, stands as a towering achievement in American literature. This epic tale of obsession, vengeance, and man's struggle against nature follows the monomaniacal Captain Ahab in his relentless pursuit of the white whale, Moby Dick. Set against the backdrop of the 19th-century whaling industry, the novel is renowned for its complex narrative structure, rich symbolism, and profound philosophical musings.
Moby Dick weaves together diverse themes including the nature of good and evil, the limits of human knowledge, and the conflict between free will and fate. Its vast cast of characters, from the narrator Ishmael to the diverse crew of the Pequod, represents a microcosm of human society. The novel's dense prose, filled with digressions on topics ranging from cetology to the nature of whiteness, has both challenged and fascinated readers for generations.
The depth and complexity of Moby Dick make it an ideal subject for advanced textual analysis with Hellixia's cutting-edge analytical features. Our approach combines the power of Large Language Models (LLMs) with advanced data visualization techniques to uncover new insights into Melville's masterpiece.
Our analysis will unfold in three distinct phases, each leveraging different capabilities of Hellixia:
-
LLM-Powered Graphical Representations: We begin by harnessing Hellixia's ability to generate graphs solely from the book's title. This phase will produce:
- Semantic Flowcharts illustrating the progression and sequence of key concepts in Moby Dick.
- A Causal Network outlining the key factors contributing to a successful narration of the novel.
-
Semantic Networks: Next, we'll combine LLM knowledge with embeddings to create semantic networks. These networks will be built around a carefully curated set of keywords designed to capture the most significant dimensions of the book.
-
Targeted Textual Analysis: Finally, we'll dive into specific passages of Moby Dick, using Hellixia to analyze the text directly. This approach will meld the studied text with the integrated knowledge of the LLMs, offering a unique perspective on Melville's words.
Join us as we navigate the complex waters of Moby Dick using Hellixia, offering fresh insights into one of literature's greatest masterpieces.
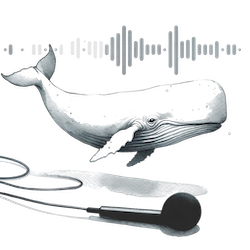
Check out the podcast automatically generated by NotebookLM.
LLM-Powered Graphical Representations
The first step in our exploration of Moby Dick involves identifying the most fundamental elements within the book. We will begin by unveiling the core ideas that shape the narrative, then progressively increase the complexity to gain more detailed insights and a deeper understanding of the novel's underlying layers.
To achieve this, we will employ various completion models and adjust complexity settings, generating multiple graphical representations. This process will demonstrate the non-deterministic nature of these models, emphasizing that no single analysis is definitive. Just as asking different human assistants can yield varied perspectives, each model offers a unique interpretation.
This approach highlights the crucial role of subject matter experts, who must carefully select, refine, and validate the generated models to ensure accuracy and relevance. By illustrating this process, we underscore the importance of human oversight in leveraging LLM-powered tools for analysis.
Semantic Flowchart - Default Settings
We present here a method for developing a Semantic Flowchart by directly leveraging the knowledge embedded in LLMs.
- We begin by creating a node titled "Herman Melville- Moby Dick."
- After selecting this node, we use Hellixia's
Semantic Flowchart Generator
feature. - We configure the settings with Claude 3.5 Sonnet as shown in the screenshot below:
Semantic Flowchart Dialog
As a result, the LLM-powered process generates the following Semantic Flowchart:
Semantic Flowchart with Claude 3.5 Sonnet - Default Settings
Employing the same analytical process, but utilizing the OpenAI o1-mini completion model instead of Claude, we generated the following Semantic Flowchart:
Semantic Flowchart with o1-mini - Default Settings
Comparing the two Semantic Flowcharts, we notice that the one generated by the OpenAI o1-mini model offers a somewhat more granular view of the book's concepts.
It's important to note that:
- Even if we were to reapply the same process with identical prompts and completion models, we would likely obtain slightly different models.
- This variability occurs due to the inherently stochastic nature of LLMs.
- Importantly, this variation persists even when the temperature is set to 0 in Hellixia.
This inherent variability in LLM outputs underscores the importance of multiple runs and expert interpretation in AI-assisted analysis. It also highlights why a single output should not be treated as definitive, but rather as one perspective in a range of possible interpretations.
Semantic Flowchart - High Complexity
Advancing our analysis, we now adjust the complexity slider to its maximum value (as illustrated below) while continuing to use the OpenAI o1-mini model.
Semantic Flowchart Dialog - High Complexity
This configuration yielded the following, more intricate Semantic Flowchart:
Semantic Flowchart with o1-mini - High Complexity
Do not hesitate to right-click on the image and open it in a new tab to zoom in.
Below is the Semantic Flowchart generated using Claude 3.5 Sonnet model with the same settings:
Semantic Flowchart with Claude 3.5 Sonnet - High Complexity
Likely due to the high complexity setting we applied, this flowchart consists of two distinct parts, with the second graph positioned in the upper right corner.
Causal Network
We now proceed with our analysis by utilizing another powerful tool in Hellixia: the Automatic Causal Network Generator. For this step, we use "Herman Melville – Moby Dick Plot" as the input question (see the dialog below).
Automatic Causal Network Generator Dialog
Hellixia then generates a Causal Graph, which outlines the key factors that contribute to crafting a successful narration of Moby Dick.
Causal Network
Semantic Network Analysis: Combining LLM Knowledge with Embeddings
We now advance our study of Moby Dick by integrating two powerful computational approaches:
- Knowledge from LLMs
- Machine learning techniques using Embeddings
Embeddings
Embeddings are numerical representations of tokens, words, or sentences that capture their meanings based on context. For tokens and words, embeddings map linguistic units to vectors, allowing the model to understand similarities and differences. Sentence embeddings extend this concept, representing entire phrases or sentences as vectors, enabling models to grasp contextual meaning and relationships in larger textual structures. These embeddings allow machines to process and compare language efficiently across various tasks.
This integrated approach enables us to learn semantic networks, offering an alternative perspective for understanding the novel's complex structure and themes.
Methodology
Our semantic networks are structured around a carefully curated set of keywords. These keywords are specifically selected to capture the most significant dimensions of Moby Dick.
Benefits
This method enables:
- A more detailed analysis of the text
- Comprehensive exploration of key elements and themes
- Visualization of relationships within the narrative
Through this approach, we aim to uncover deeper insights into Melville's masterpiece, revealing dimensions that are not apparent in the previous analyses we've carried out.
Characters Analysis
We begin our semantic network analysis by focusing on the main characters.
For this, we utilize Hellixia's Automatic Semantic Network Generator
, specifically using its Advanced Mode to select Characters
as the focal point from the 163 available keywords.
Automatic Semantic Network Generator Dialog
Semantic Network of the Main Characters
This semantic network, generated using the Maximum Weight Spanning Tree
algorithm, represents the strongest correlations between the semantics associated with the characters' names and their descriptions.
Comment Development
To deepen our understanding of the characters, we leverage Hellixia's newly integrated features, which allow us to develop or summarize the comments associated with selected nodes (accessible via Node Contextual Menu > Properties > Comments
).
This ability to expand and condense comments offers remarkable flexibility in our exploration of the novel.
When a more in-depth analysis is required, we can develop these comments to access detailed and contextualized explanations.
Node Comment Elaborator Dialog
Semantic Network of the Main Characters with Extended Comments
If we want a more concise overview, we can, of course, summarize the elaboration generated previously.
Node Comment Condenser Dialog
Semantic Network of the Main Characters with Condensed Comments
Weight of the Characters
Now, suppose we want to assign a weight reflecting the importance of each character in the book. We can use the query "Herman Melville – Moby Dick (Add a weight in brackets from 0 to 100 to each node's comments to reflect the importance of that dimension)" to achieve this.
Semantic Network of the Main Characters with their Relative Importance
Once again, we observe the non-determinism of LLMs. Adding weights results in a slightly different list of characters.
Predefined Group: Book Analysis
Let's continue our analysis with the generation of a semantic network based on the predefined group Book Analysis
, composed of the following 10 carefully selected keywords designed to capture the most important dimensions of books:
- Characteristics
- Concepts
- Contributions
- Elements
- Ideas
- Impacts
- Perspectives
- Progressions
- Purposes
- Themes
Automatic Semantic Network Generator Dialog
Book Analysis Semantic Network
Node Force Analysis
Below, we have the same semantic network, but represented with the mapping of the Node Force Analysis
.
Book Analysis Semantic Network - Node Force Mapping
Variable Clustering
We now conclude this semantic network analysis part by using BayesiaLab's Variable Clustering
algorithm to create group of variables that are strongly correlated.
We use the settings below, where we use Hellixia to automatically create a name for each cluster of variables, based on the variables belonging to the cluster.
Variable Clustering Dialog
Book Analysis Semantic Network - Clusters of Variables
This graph can be seen as a macroscopic view of the previous node force mapping.
Textual Analysis
The final part of this analysis of Moby Dick consists of using Hellixia to analyze specific excerpts from the text. We will begin with a simple paragraph and then proceed to a more in-depth analysis of Chapter 9 (The Sermon) and 42 (The Whiteness of the Whale).
Key Passage Analysis from Chapter 132
Is it I, God, or who, that lifts this arm? But if the great sun move not of himself; but is as an errand-boy in heaven; nor one single star can revolve, but by some invisible power; how then can this one small heart beat; this one small brain think thoughts; unless God does that beating, does that thinking, does that living, and not I.
We begin by creating a node in a new graph and pasting the selected paragraph into the comment section of the node.
Once the node is selected, we utilize Hellixia’s Semantic Flowchart Generator
feature.
The settings are configured using Claude 3.5 Sonnet, as demonstrated in the screenshot below:
Semantic Flowchart Generator Dialog
As a result, the LLM-powered process generates the following Semantic Flowchart:
Semantic Flowchart Generated on the Node Comment
We also utilize this paragraph to demonstrate the Causal Semantic Diagram
feature, which focuses on representing the cause-and-effect relationships between key concepts:
Causal Semantic Diagram Generated on the Node Comment
Analysis of Chapter 9 – The Sermon
Now, instead of focusing on just a paragraph, we will expand our analysis to cover an entire chapter—The Sermon.
We begin by copying the entire chapter into a text file, which will serve as a knowledge file for analysis.
Next, we utilize the Document Analysis > Semantic Flowchart Generator
feature, applying the parameters listed below:
Semantic Flowchart Generator Dialog
This method automatically generates Semantic Flowcharts based on the content of Chapter 9, enabling a clear visualization and deeper understanding of its key concepts.
Causal Semantic Diagram Generated on Chapter 9 with Gemini 1.5 pro
The percentages indicated in the comments of the nodes represent the approximate location of the concepts within the knowledge file. This feature allows for a visualization of the distribution of key ideas throughout the analyzed text.
Analysis of Chapter 42 – The Whiteness of The Whale
We conclude this analysis with Chapter 42: The Whiteness of The Whale.
Following the same workflow used for Chapter 9, we generate the flowchart—this time using Claude 3 Opus instead of Gemini 1.5 Pro.
Causal Semantic Diagram Generated on Chapter 42 with Claude 3 Opus
Finally, we create one last flowchart, adjusting the complexity to Medium to generate a slightly more detailed representation.
Causal Semantic Diagram Generated on Chapter 42 with Claude 3 Opus - Medium Complexity
Conclusion
In conclusion, our analysis of Moby Dick using Hellixia's advanced features demonstrates the power of modern tools in deepening our understanding of literary works.
From the creation of semantic flowcharts to the exploration of causal relationships, Hellixia enables a fresh and structured perspective on Melville's narrative.
The machine learning of semantic networks, using dimensions extracted with a carefully selected set of keywords designed to capture the most important aspects of the book, allows us to uncover deeper insights into the novel, revealing new dimensions.
Finally, Hellixia also allows us to deep dive into specific excerpts of the book, creating flowcharts or causal semantic diagrams for detailed analysis.