Imputation
Context
- The Imputation function allows you to assign permanent values for the Missing Values in the dataset that is associated with your Bayesian network.
- This Imputation permanently replaces the estimated missing value distributions, which BayesiaLab maintains as placeholders for the missing values in the dataset.
Usage
- In the Modeling Mode, select one or more nodes, of which at least one node contains Missing Values.
- Node Context Menu > Imputation.
- The Choose Imputation dialog box opens up, which features a range of options.
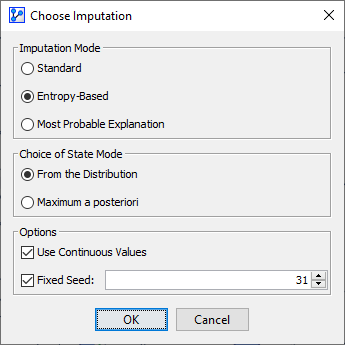
Imputation Mode
Standard
Within a data record, the Standard Imputation Mode randomly chooses the sequence of nodes for which missing values are imputed.
Entropy-Based
The Entropy-Based Imputation Mode selects the imputation order within a record based on the conditional entropy of the nodes with missing values.
More specifically, the imputation order within a record is determined according to the following two criteria:
- Nodes that have a fully observed (or already-imputed) Markov Blanket are imputed first.
- Then, the imputation of nodes within a record is ordered from low to high according to their respective conditional entropy given the observed values and the already-imputed values. This means that, within each record, values of nodes with a low uncertainty are imputed before nodes with high uncertainty.
Most Probable Explanation
- This Imputation Mode is based on the Maximum A Posteriori (MAP) Query.
- Given the observed values in a record, the MAP Query identifies those states of the nodes with missing values that maximize the joint probability of the record.
- The set of states identified by the MAP Query are then imputed for the missing values in the record.
- As the MAP Query determines all the joint-probability-maximizing states in a record at the same time, there is no order to the imputation sequence.
Choice of State Mode
From the Distribution
- The missing values of a node are imputed by randomly drawing values from the posterior probability distribution of the node given the values of all the other nodes.
- With this approach, the variance of a node's distribution remains the same before and after the imputation.
- However, by drawing values from the posterior probability distribution, individual records may not be imputed with the most probable value for that record.
- In that sense, this approach is not suitable for record-level prediction or scoring.
- This imputation method is appropriate if you have to impute values and plan to use the imputed dataset for further learning or wish to compute statistics for the imputed nodes.
Maximum a posteriori
- The values are imputed deterministically based on the posterior distribution of each node given the values of all the other nodes.
- This imputation policy is optimal at the record level, i.e., it assigns the most probable value for each missing value.
- So, if the imputed dataset is meant to be utilized and interpreted at the record level, this is the appropriate approach.
- However, a major drawback of this method is that the imputed node's distribution is no longer the same as before the imputation, i.e., the node's statistics would change.
Once the imputation is performed, there is no way to remove the imputed values and revert to the original dataset that included the Missing Values.