Car Accidents
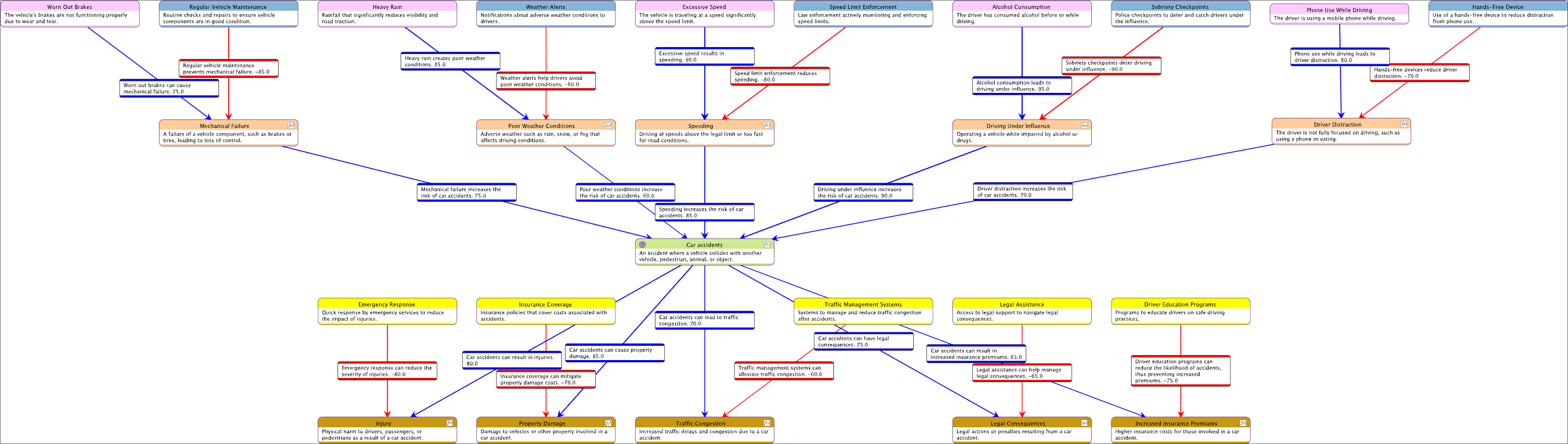
Risk-Centric Causal Network on Car Accidents
Car accidents are multifaceted events influenced by a wide range of factors, from driver behavior to environmental conditions. Gaining a comprehensive understanding of these causes and risks is essential for developing targeted prevention strategies. Hellixia offers two powerful approaches for constructing Causal Bayesian Networks that provide deep insights into these complex dynamics.
-
Causal Mechanism Network: This method constructs a causal network that explains the underlying mechanisms contributing to a specific criterion, such as the probability of a car accident. By identifying direct and indirect causal factors, this approach helps to reveal the interactions and probabilities that drive accident occurrence.
-
Risk-Centric Causal Network: The second approach focuses on a Causal-Centric view, where the chosen criterion (e.g., car accidents) is treated as the main risk. This approach organizes the analysis around five distinct groups of dimensions:
- Events: Sub-risks associated with the main risk (car accidents), detailing specific situations that may lead to accidents.
- Triggers: Variables that initiate Events, identifying what might cause sub-risks to manifest.
- Controls: Factors that can prevent or reduce the probability of Triggers causing Events.
- Consequences: Direct impacts of Events, such as injuries or property damage resulting from car accidents.
- Mitigants: Variables that lessen or mitigate the severity of Consequences.
Both approaches provide a detailed understanding of the factors at play in car accidents, offering engineers and researchers a robust framework for analyzing and reducing accident risks. In this article, we will explore how Hellixia leverages these methods to create Causal Bayesian Networks and Risk-Centric Causal Bayesian Networks, guiding decisions and safety interventions.
Causal Bayesian Network
First, we go to the BayesiaLab Startup Page and select the Automatic Causal Network Generator tool.
BayesiaLab Startup Page
Next, we enter the main topic Car Accidents (for example, using the microphone ) and select Advanced Mode.
Automatic Causal Network Generator Dialog
After selecting the completion model (in this case, Claude Sonnet), we choose a low complexity setting, include consequential variables, and enable the estimation of prior probabilities for the root nodes. Within seconds, we obtain a fully specified Causal Bayesian network, complete with both the graph and probabilities.
This network consists of Boolean nodes and directed arcs that represent causal relationships. It displays 5 events (orange nodes), 3 interventions (green nodes), 2 aggregates (yellow nodes), 1 confounding variable (Time of day), and 3 consequential variables (blue nodes). Each arc includes a brief explanation of its causal link, along with an estimated effect, ranging from -100 (displayed in red) to 100 (displayed in blue). The negative and positive effects are incorporated into the DualNoisyOr() function of the child nodes. Additionally, each root node has an estimated prior distribution.
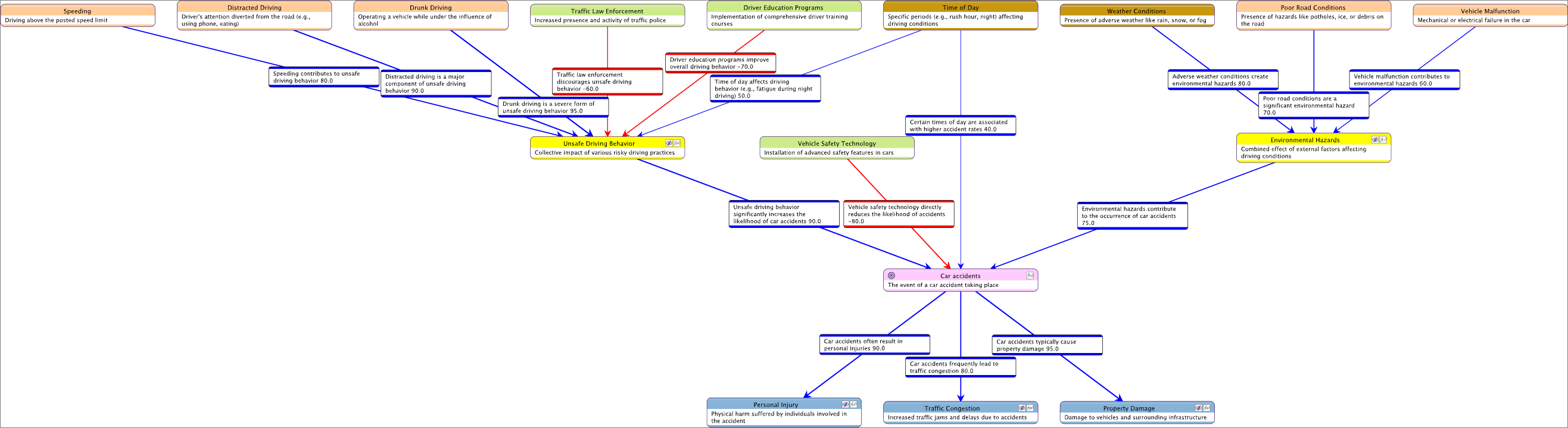
Causal Bayesian Network on Car Accidents
The Tornado graph (Analysis > Visual > Target > Target's Posterior > Tornado Diagrams > Total Effect
) below illustrates the impact of events and intervention variables on the probability of a car accident.
Tornado Plot on Car Accidents
Risk-Centric Causal Bayesian Network
We will now create a risk-centric causal network, with car accidents considered as the main risk.
We go to the BayesiaLab Startup Page, select the Automatic Causal Network Generator tool, and then select Advanced Mode to check the Risk-Centric option.
Automatic Risk-Centric Causal Network Generator Dialog
The network below displays the generated structure, which includes triggering variables (pink nodes), control variables (blue nodes), events (orange nodes), consequences of car accidents (brown nodes), and mitigants (yellow nodes).
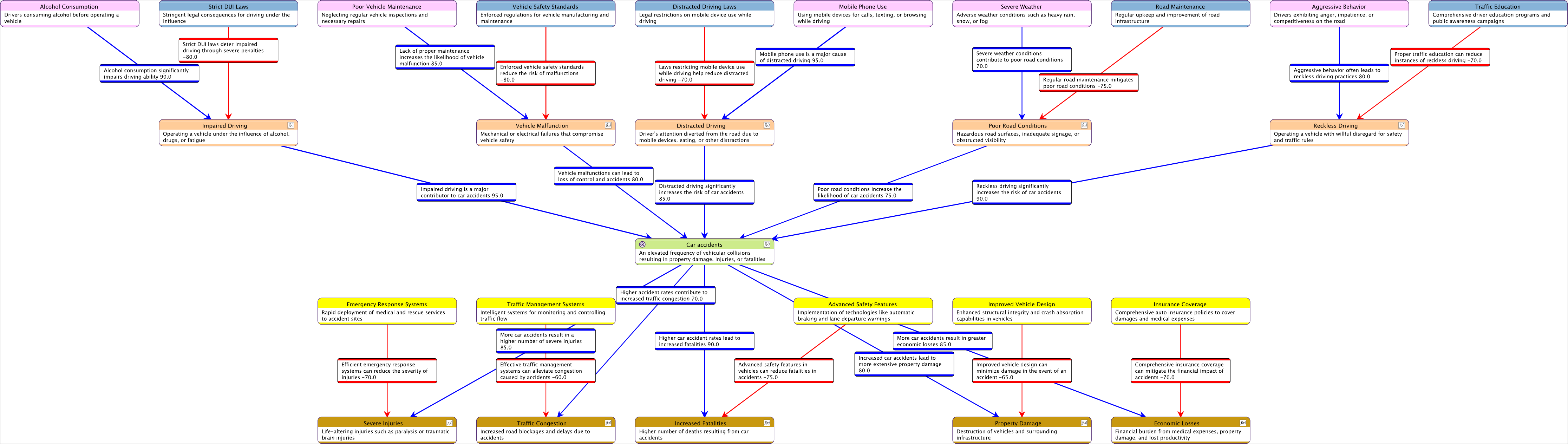
Risk-Centric Causal Bayesian Network on Car Accidents
We then generate the Relationship Analysis report (Analysis > Report > Relationship
) to assess the strength of the relationships between the nodes and use Hellixia to summarize the arc and node forces within this network.
Relationship Analysis Report
Dialog for generating the Summary of the Relationship Analysis Report
Summary of the Relationship Analysis Report