Arc Confidence
The menu item Tools>Cross validation>Arc confidence allows computing a frequency value that measures the robustness of each arc. The network needs a database, and the Validation Mode must be activated.
For this purpose, the Jackknife method is used. It consists of splitting the database into k samples of equal size but before this step, the database is shuffled in order to avoid bias due to a sorted database. In this context, k-1 samples are used in order to learn k networks while the k sample is not used.
If the initial database is stratified then the generated database will be stratified in the same way.
The structural coefficients are updated for the new networks in the following way:
where:
- is the structural coefficient of the current network,
- is the sum of the weights of all the observations described in the data set
- is the sum of the weights of the observations contained in the sub data set
Parameters
In the following window, the learning algorithm shall be selected, as well as the number of data samples to be generated.
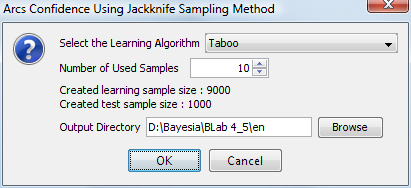
The window displays sample size depending on the size of the database and the number of samples. The samples are chosen randomly in order to avoid the errors that can occur when the database is sorted.
In the output directory can be saved all networks and databases learnt during Jackknife process.
Depending on the chosen learning algorithm, a dialog box displays specific settings :
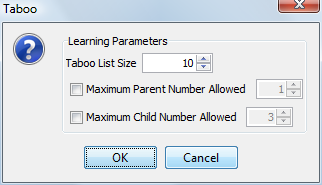
Analysis Report
Once the networks have been learnt on each data sample, the following report is displayed :
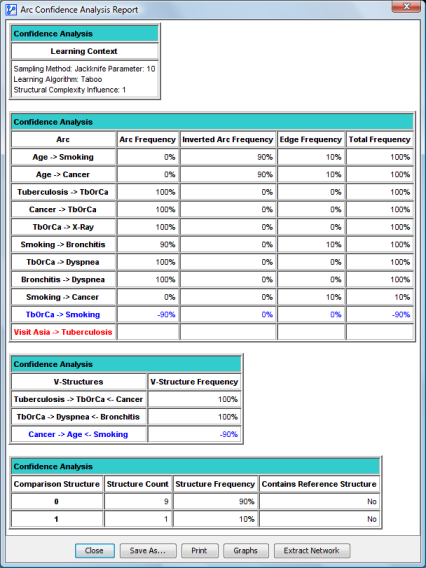
It is composed of four parts :
- The learning context: Reminds the learning method and the number of data samples. It also indicates the structural complexity coefficient used (it is the same as the one used in the initial network).
- Arcs confidence analysis: Lists of arcs grouped by three colored types
- Black: the arcs that exist both in the reference structure and the networks from the samples.
- Arc frequency represents how often the arc appeared with the same orientation as in the sample networks.
- Inverted arc frequency represents how often the arc appeared with the reverse orientation as in the sample networks.
- Edges frequency represents how often the arc appeared without any orientation as in the sample networks (the equivalence class of the learnt networks is used).
- Total frequency is the sum of all previous ones. It indicates the overall strength between the variables.
- Blue: the arcs that exist in at least one sample network but that do not exist in the reference network. In this case, the frequencies are displayed with a negative value. The reference orientation of arc is arbitrarily given by the first arc found in the first sample network.
- Arcs frequency represents how often this arc appeared with the same orientation as the first.
- Inverted arc frequency represents how often this arc appeared with the reverse orientation compared with the first.
- Edge frequency represents how often the arc appeared without any orientation (equivalence class).
- Total frequency is the sum of all previous ones. It indicates the overall strength of a relationship that does not exist in the reference network.
- Red: arcs that exist in the reference structure but that have never been found in any learnt sample.
- Black: the arcs that exist both in the reference structure and the networks from the samples.
- V-Structures confidence analysis: Lists of V-Structures grouped by three colored types:
- Black: V-Structures that exist both in the reference structure and the networks from the samples.
- Blue: V-Structures that do not exist in the reference structure but that appeared in at least once in a sample network. Frequencies are displayed with a negative value.
- Red: V-Structures that exist in the reference network but that never appeared in any sample network.
- Comparison structure array: It summarizes all learnt networks from the samples. When identical structures exist, they are gathered.
- First column is the structure identifier
- The second column is the number of identical structures learnt.
- The third column represent the frequency of the whole structure: it is the number of times the current structure appears divided by the total number of structures.
- The last column indicates whether the reference structure is included or not in the current structure.
The report can be saved in a HTML format file. It can also be printed. Two other options exist: displaying graphs and extracting the network.
Graphs
The Structure Comparison button from the report allows displaying the graphical structure comparator. With this tool, data contained in reports can be viewed and interpreted easily.
Extracting the Network
The Network extraction button from the report displays network extraction tool. This tool allows building a network from any structure depending on arcs frequency thresholds.