Targeted Cross Validation
Allows performing a targeted cross validation based on the current network.
The requirements are:
- the network must have associated data,
- the validation mode must be activated and
- the network must have a target variable.
For targeted cross validation, the k-Folds method is used. It consists at splitting the database into k parts (the folds) and using k - 1 folds for learning a set of k networks but before this step, the database is shuffled in order to avoid bias due to a sorted database. For each network, the last k-th fold is used for testing the network and measuring its predictive performance. For each network learnt, the continuous variables are re-discretized according to the variable distribution in the fold. The discretization method is the same as the one used for the reference network. On the contrary, initial aggregations are kept.
If the initial database is stratified then the generated database wil be stratified in the same way.
The structural coefficients are updated for the new networks in the following way:
where:
- is the structural coefficient of the current network,
- is the sum of the weights of all the observations described in the data set
- is the sum of the weights of the observations contained in the sub data set
On this basis, the networks structures are learnt using the chosen algorithm, and the network's targeted network performance is computed.
Parameters
The learning algorithm and the number of folds shall be chosen from this dialog box:
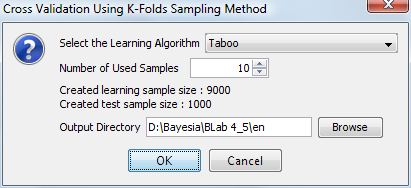
The sample size is calculated depending on the size of the database and the number of folds. The samples are chosen randomly in order to avoid the errors that can occur when the database is sorted.
An output directory can be specified where all intermediate networks learnt from the folds shall be saved with their corresponding database.
Results
Network's targeted performance is displayed in this window:
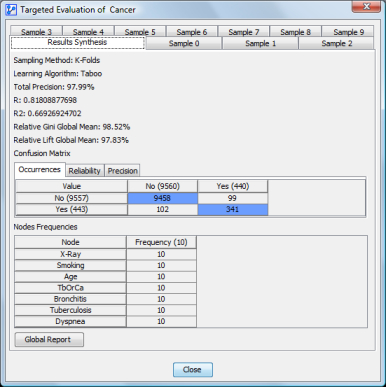
The first panel "results synthesis" displays the global results computed on all the samples for:
- global precision
- R: Pearson's coefficient
- R2: squared Pearson's coefficient
- relative Gini value
- relative Lift value
- confusion matrices : for occurrences, for reliability and for precision
Nodes frequency array indicates how often a node appears in any network built upon a fold (no matter it is directly connected to the target node or not).
The Global report button (in the synthesis panel) displays the cross validation synthesis report.
The tabs contain the targeted performance result of each network learnt on the folds:
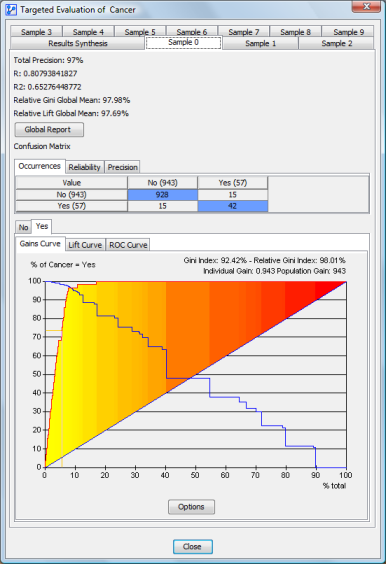
The details about the panel contents can be found in the targeted performance targeted performance report section.
Global Analysis Report
Once all the networks are learnt, the following report is generated:
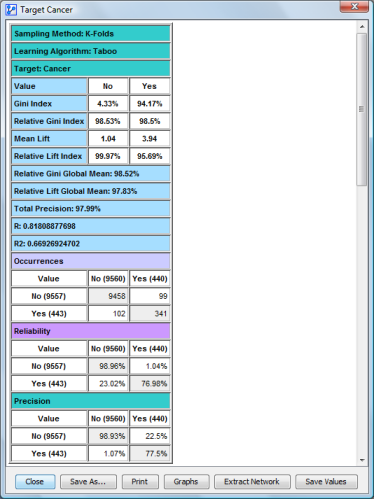
The report is built on the same template as the global targeted evaluation report, save that it summar- izes all values for each index and each matrix calculated for each fold.
The rest of the report contains the nodes frequencies.
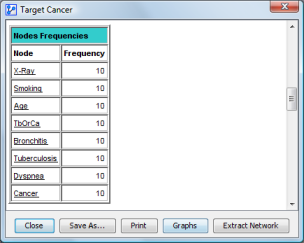
The last part of the report contains structural comparison of reference network with the generated net- works :
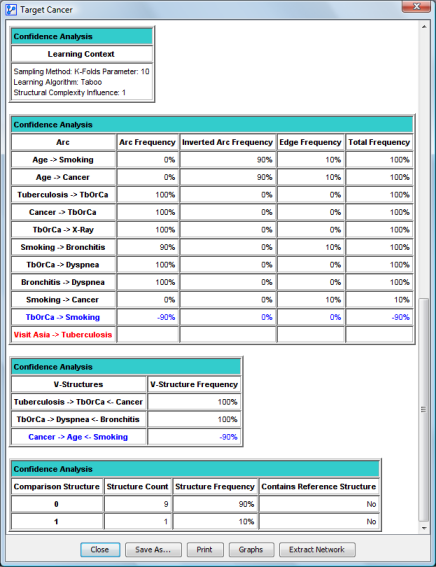
Its contents are the same as arcs confidence analysis.
This report can be saved in a HTML-format file and can also be printed. Two other options exist: displaying graphs and extracting the network.
Graphs
The Graphs button from the report allows displaying the graphical structure comparator. With this tool, data contained in reports can be viewed and interpreted easily.
Extracting the Network
The Network extraction button from the report displays network extraction tool. This tool allows building a network from any structure depending on arcs frequency thresholds.
Saving the Values
The Save Values button of the report allows saving into a file the numerical values of the target predicted by each network on its corresponding test set.