Probabilistic Evidence
Context
- Probabilistic Evidence is a convenient way for directly encoding our assumptions about possible conditions of a domain.
- For example, a stock market analyst may consider a scenario with a specific probability distribution for JNJ corresponding to a hypothetical period of time (i.e., a subset of days).
- Given his understanding of the domain, he can assign probabilities to each state, thus encoding his belief.
Usage
-
After removing the prior evidence, we can set such beliefs as Probabilistic Evidence by right-clicking the JNJ Monitor and then selecting
Enter Probabilities
. -
For the distribution of Probabilistic Evidence, the sum of the probabilities must be equal to 100%.
-
We can adjust the Monitor’s bar chart by dragging the bars to the probability levels that reflect the scenario under consideration. By double-clicking on the percentages, we can also directly enter the desired probabilities. Note that changing the probability of any state automatically updates the probabilities of all other states to maintain the sum constraint.
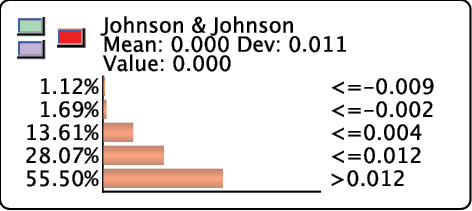
- In order to remove a degree of freedom in the sum constraint, we left-click the State Name/Value in the Monitor, to the right of each bar.
- Doing so locks the currently set probability and turns the corresponding bar turns green.
- The probability of this state will no longer be automatically updated while the probabilities of other states are being edited. This feature is essential for defining a distribution on nodes that have more than two states.
- Another left-click on the same State Name/Value unlocks the probability again.
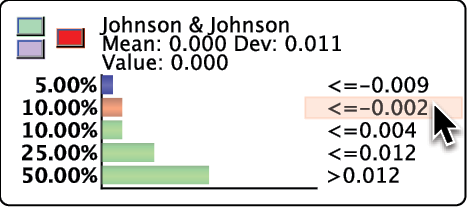
Observation Type
- There are two ways to validate the entered distribution, via the green and the purple buttons.
- Clicking the green button () defines a static likelihood distribution.
- This means that any additional piece of evidence on other nodes can update the distribution we set.
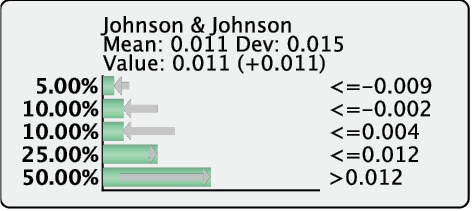
- Clicking the purple button () “fixes” the probability distribution we entered by defining dynamic likelihoods.
- This means that each new piece of evidence triggers an update of the likelihood distribution in order to maintain the same probability distribution.
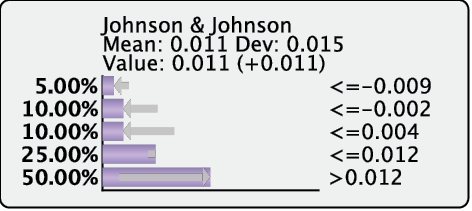
- Using either validation method, BayesiaLab computes a likelihood distribution that produces the requested probability distribution. By setting this distribution, BayesiaLab also performs inference automatically and updates the probabilities of the other nodes in the network.
