New Three-Day Course: Harnessing the Power of Generative AI with Hellixia
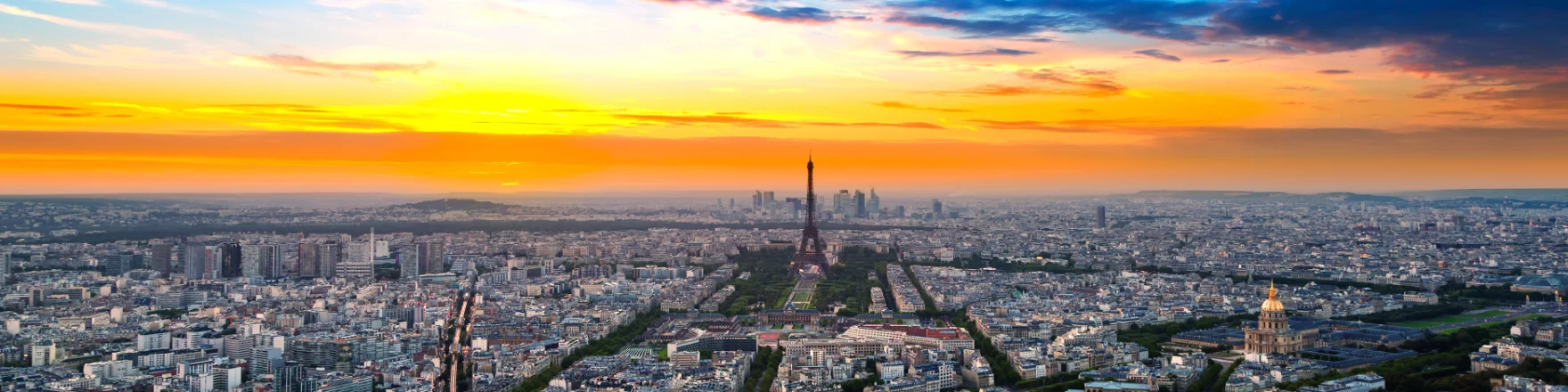
June 10-12, 2025
Mitwit Paris Gare Montparnasse
46 bis, avenue du Maine, 75015 Paris, France
Course Overview
This new three-day course program is designed to help you employ Generative AI in the context of Bayesian networks using Hellixia, BayesiaLab's GenAI assistant. Hellixia offers a powerful set of capabilities to streamline the design, analysis, and documentation of knowledge models.
The course focuses on the five core functions of Hellixia, giving you practical skills to integrate them into your modeling workflows. These core functions either use internal knowledge embedded in Large Language Models (LLMs) or combine it with specific knowledge files, similar to Retrieval-Augmented Generation (RAG).
1. LLM Knowledge Mining
LLM Knowledge Mining taps into the knowledge embedded in Large Language Models (LLMs) and can automatically generate a wide variety of network types, including:
- Semantic Networks
- Semantic Flowcharts
- Causal Semantic Diagrams
- Knowledge Graphs
- (Risk) Causal Networks.
2. LLM-Augmented Machine Learning
LLM-Augmented Machine Learning can identify causal relationships between nodes and automatically add arcs to a network and/or propose Structural Priors. Hellixia also generates narratives to explain these relationships for easier interpretation and subsequent validation by domain experts.
In this context, Hellixia can also propose meaningful names for newly-induced factors (latent variables) generated by BayesiaLab's Multiple-Clustering function. Similarly, Hellixia can suggest names for segments identified with BayesiaLab's Data Clustering function.
3. LLM-Powered Brainstorming Assistants
LLM-Powered Brainstorming Assistants support and accelerate brainstorming sessions by leveraging dimensions provided by subject matter experts (e.g., via (BEKEE)[/bekee]). Hellixia uses these inputs to construct a semantic network that organizes, clusters, and defines the dimensions, thereby becoming a crucial tool for deciding which dimensions to include in a model.
In the quantitative phase, Hellixia assists in the elicitation of Root Priors and ICI Local Effects, providing probability values, confidence levels, and explanatory texts.
4. LLM Text-Driven Causal Discovery
The LLM Text-Driven Causal Discovery function analyzes unstructured textual data — such as customer reviews, transcripts, survey responses, or knowledge documents — to extract structured insights from LLMs. Hellixia identifies key drivers and themes, organizes them into a semantic network, and elicits Root Priors and ICI Local Effects for each dimension, thus enabling a full driver analysis and a subsequent optimization. This mirrors the brainstorming workflow with subject matter experts (see (BEKEE)[/bekee]) but relies entirely on LLM intelligence, making it ideal when expert input is unavailable or when handling large volumes of freeform text.
5. LLM-Enhanced Network Documentation Tools
LLM-Enhanced Network Documentation Tools simplify and enrich the documentation and presentation of networks. Hellixia generates Node Comments, Long Names, and narratives about relationships. Furthermore, it supports the multilingual translation of networks, and creates icons for nodes based on their semantic content.
Course Software
If you currently have BayesiaLab license, it automatically includes Hellixia, and you can use it for the course. If you don't a BayesiaLab license, there is no need to get one specifically for the course. We will provide you with a BayesiaLab Continental Token license, valid for two months, which you can use during the course and for your own independent practice afterwards.
Additionally, all participants will receive an AI Power Pack (opens in a new tab), valid for two months, providing you with all the API keys that are necessary for Hellixia to access Large Language Models, including OpenAI, Anthropic, Google, Mistral, and Deepseek.
About the Instructor
- Dr. Lionel Jouffe is co-founder and CEO of France-based Bayesia S.A.S. Lionel holds a Ph.D. in Computer Science from the University of Rennes and has worked in Artificial Intelligence since the early 1990s. While working as a Professor/Researcher at ESIEA, Lionel started exploring the potential of Bayesian networks.
- After co-founding Bayesia in 2001, he and his team have been working full-time on the development of BayesiaLab. Since then, BayesiaLab has emerged as the leading software package for knowledge discovery, data mining, and knowledge modeling using Bayesian networks. It enjoys broad acceptance in academic communities, business, and industry.
FAQ
What is the course format?
The course is an instructor-led classroom-based program with a maximum of 15 participants. The small group size allows for one-on-one coaching during the hands-on exercises and facilitates a lively dialog between participants.
Who should attend?
Applied researchers, statisticians, data scientists, data miners, decision scientists, biologists, ecologists, environmental scientists, epidemiologists, predictive modelers, econometricians, economists, market researchers, knowledge managers, marketing scientists, operations researchers, social scientists, students and teachers in related fields.
What background is required?
- Basic data manipulation skills, e.g., with Excel.
- No prior knowledge of Bayesian networks is required.
- No programming skills are required. You will use the graphical user interface of BayesiaLab for all exercises.
- For a general overview of this field of study, we suggest you read our free e-book, Bayesian Networks & BayesiaLab. Although not mandatory, reading its first three chapters would be an excellent preparation for the course.
Can I attend this course remotely?
No, this is an in-person course, and you will need to be present in the classroom.
What do I need to bring?
- You must bring your own notebook or laptop computer running a 64-bit version of Windows or macOS. For macOS computers, BayesiaLab is compatible with both Intel and Apple Silicon.
A tablet-type iOS or Android computer cannot run BayesiaLab and will not work for this course.
-
Before the course, you will receive download and activation instructions for BayesiaLab so that your setup is ready to go when the course starts.
-
A mouse as a pointing device is strongly recommended.
What is the cancellation and refund policy?
You may cancel your registration for a full refund of the course fees up to 30 days before the start of the course. If you cancel within 30 days of the event, your course fee will not be refunded. However, you will be able to apply 100% of the paid course fees towards future BayesiaLab courses